Long-Context LLMs Meet RAG
18 ott 2024 ·
15 min. 35 sec.
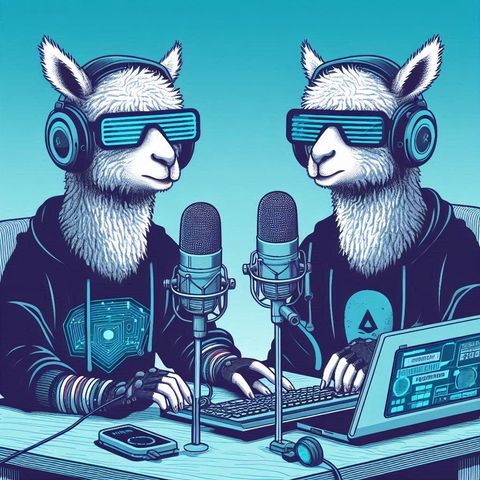
Scarica e ascolta ovunque
Scarica i tuoi episodi preferiti e goditi l'ascolto, ovunque tu sia! Iscriviti o accedi ora per ascoltare offline.
Descrizione
📈 Long-Context LLMs Meet RAG: Overcoming Challenges for Long Inputs in RAG This paper explores the challenges and opportunities of using long-context language models (LLMs) in retrieval-augmented generation (RAG) systems....
mostra di più
📈 Long-Context LLMs Meet RAG: Overcoming Challenges for Long Inputs in RAG
This paper explores the challenges and opportunities of using long-context language models (LLMs) in retrieval-augmented generation (RAG) systems. While increasing the number of retrieved passages initially improves performance, the authors find that it eventually degrades due to the introduction of irrelevant information, or "hard negatives." To address this, the paper proposes three methods for enhancing the robustness of RAG with long-context LLMs: retrieval reordering, RAG-specific implicit LLM fine-tuning, and RAG-oriented LLM fine-tuning with intermediate reasoning. The paper also investigates the impact of various factors related to data distribution, retriever selection, and training context length on the effectiveness of RAG-specific tuning.
📎 Link to paper
mostra meno
This paper explores the challenges and opportunities of using long-context language models (LLMs) in retrieval-augmented generation (RAG) systems. While increasing the number of retrieved passages initially improves performance, the authors find that it eventually degrades due to the introduction of irrelevant information, or "hard negatives." To address this, the paper proposes three methods for enhancing the robustness of RAG with long-context LLMs: retrieval reordering, RAG-specific implicit LLM fine-tuning, and RAG-oriented LLM fine-tuning with intermediate reasoning. The paper also investigates the impact of various factors related to data distribution, retriever selection, and training context length on the effectiveness of RAG-specific tuning.
📎 Link to paper
Informazioni
Autore | Shahriar Shariati |
Organizzazione | Shahriar Shariati |
Sito | - |
Tag |
Copyright 2024 - Spreaker Inc. an iHeartMedia Company