Inference Scaling for Long-Context RAG
20 ott 2024 ·
12 min. 17 sec.
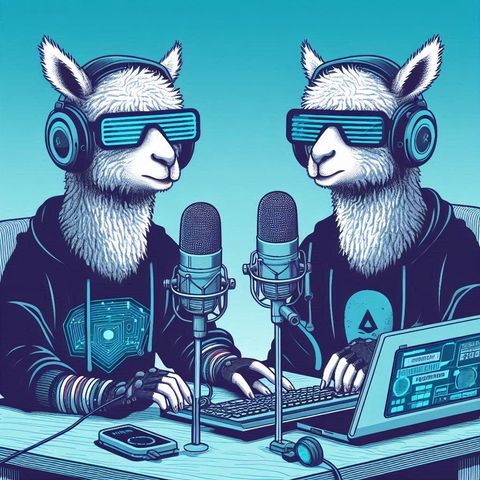
Scarica e ascolta ovunque
Scarica i tuoi episodi preferiti e goditi l'ascolto, ovunque tu sia! Iscriviti o accedi ora per ascoltare offline.
Descrizione
🗓 Inference Scaling for Long-Context Retrieval Augmented Generation This research paper explores the effectiveness of inference scaling for retrieval augmented generation (RAG), a technique that enhances large language models (LLMs)...
mostra di più
🗓 Inference Scaling for Long-Context Retrieval Augmented Generation
This research paper explores the effectiveness of inference scaling for retrieval augmented generation (RAG), a technique that enhances large language models (LLMs) by incorporating external knowledge. The authors introduce two strategies, demonstration-based RAG (DRAG) and iterative demonstration-based RAG (IterDRAG), for effectively scaling inference computation. They demonstrate that increasing inference computation, when optimally allocated, leads to nearly linear gains in RAG performance. Furthermore, they develop a computation allocation model to predict the optimal test-time compute allocation for various tasks and scenarios, showcasing its effectiveness in achieving performance gains and aligning with experimental results.
📎 Link to paper
mostra meno
This research paper explores the effectiveness of inference scaling for retrieval augmented generation (RAG), a technique that enhances large language models (LLMs) by incorporating external knowledge. The authors introduce two strategies, demonstration-based RAG (DRAG) and iterative demonstration-based RAG (IterDRAG), for effectively scaling inference computation. They demonstrate that increasing inference computation, when optimally allocated, leads to nearly linear gains in RAG performance. Furthermore, they develop a computation allocation model to predict the optimal test-time compute allocation for various tasks and scenarios, showcasing its effectiveness in achieving performance gains and aligning with experimental results.
📎 Link to paper
Informazioni
Autore | Shahriar Shariati |
Organizzazione | Shahriar Shariati |
Sito | - |
Tag |
Copyright 2024 - Spreaker Inc. an iHeartMedia Company